Daniel Kuhn
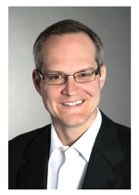
Data-Driven Distributionally Robust Optimization Using the Wasserstein Metric
Abstract: We consider stochastic programs where the distribution of the uncertain parameters is only observable through a finite training dataset. Using the Wasserstein metric, we construct a ball in the space of probability distributions centered at the uniform distribution on the training samples, and we seek decisions that perform best in view of the worst-case distribution within this ball. We show that the resulting optimization problems can be solved efficiently and that their solutions enjoy powerful out-of-sample performance guarantees on test data. The wide applicability of this approach is illustrated with examples in portfolio selection, uncertainty quantification, statistical learning and inverse optimization.
Daniel Kuhn holds the Chair of Risk Analytics and Optimization at EPFL. Before joining EPFL, he was a faculty member at Imperial College London (2007-2013) and a postdoctoral researcher at Stanford University (2005-2006). He received a PhD in Economics from the University of St. Gallen in 2004 and an MSc in Theoretical Physics from ETH Zurich in 1999. His research interests revolve around robust optimization and stochastic programming.
Esko Turunen
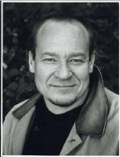
Connecting paraconsistent and many-valued logic in decision making task
Abstract: Paraconsistent logic refers to non-classical systems of logic, which reject the Principle of explosion; once a contradiction has been asserted, any proposition can be inferred. The primary motivation for paraconsistent logic is the conviction that it ought to be possible to reason with inconsistent information in a controlled and discriminating way. The principle of explosion precludes this, and so must be abandoned. The key note is evidence; if a statement is true, then there is also some evidence in favor of it, while the converse does not necessarily hold. If there is evidence in favor of a statement, it need not be true; there may also be evidence against it. Paraconsistent logics make it possible to reason with inconsistent information. Many-valued or fuzzy logics in turn are logic calculus in which there are more than two truth-values. Classical two-valued logic may be extended to infinite-valued Ćukasiewicz logic and its extension, called Pavelka logic, has infinitely many truth-values and provability degrees. We show how paraconsistency and many-valuedness can be combined in Pavelka’s logic framework. Moreover, we show a real life application in decision-making how this logic system can be utilized.
Professor Esko Turunen earned his PhD in applied mathematics, entitled `A Mathematical Study of Fuzzy Logic: an Algebraic Approach´ in 1994 at Lappeenranta University of Technology, Finland. At present Turunen is the head of the Department of Mathematics at Tampere University of Technology, Finland. His scientific interests and research areas are in many-valued logics, fuzzy logic, paraconsistent logic, data mining and their real life applications. Turunen has published more than 50 scientific articles and conference papers with peer review process including three books. Turunen has wide international contacts to several universities all over the world; he has spent more than ten years in various universities and research institutes including Charles University and Prague University of Technology in Czech Republic, University of Naples, Salerno and Pisa in Italy and Technical University in Vienna, Austria. Turunen has supervised several doctoral theses and is a member of many editorial boards of scientific journals. Turunen has represented Finland in three COST Action research projects and is currently the representative of his country in COST Action IC1406. In addition to theoretical mathematical research, Turunen has worked as a mathematical expert in many industrial research projects, such as a developer of intelligent traffic systems, medical expert systems designer and creator of various other control systems.
Carlos López Molina
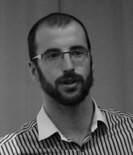
Ambiguity and Hesitancy in Quality Assesment: The case of Image segmentation
Abstract: Automatic information processing regularly deals with plenty of sources of uncertainty. Many of them are born from the data gathering itself, while many others stem from imprecise computations or algorithmic needs. In general, they can all be solved with advanced machinery, dedicated mathematical models or extra computational resources. In this effort, Fuzzy Set theory has played a relevant role in the past 40 years. However, there is a source of ambiguity and hesitancy which cannot be removed from information processing: that due to the ambiguous, human-like definition of information processing problems.
Human beings generally make variable interpretation of the goals and needs of an information processing task, whichever context it is carried out in. Hence, the perceived quality of one single result will be heterogeneous, depending on the human expert evaluating it. This poses significant problems in various stages of information processing. For example, it is damaging when it comes to algorithmic setting or optimization, since the perceived improvement (for one human) might be coupled to the perceived quality loss (according to another human). Also, it becomes damaging when scientists intend to select the best performing algorithm for a given task, as the opinions by different human experts might differ. In general, we find that the perceived quality of one single result is a conglomerate of opinions, often hesitant or contradictory.
The problem of ambiguous data for quality assessment is common in Image Processing. One clear example is the task of Image segmentation, which lacks a mathematical definition, and hence automatic methods are bound to be evaluated according to how similar their results are to human-made solutions. Unfortunately, different humans routinely produce different interpretations of an image. As a consequence, the evaluation of a segmented image becomes some sort of comparison with a list of human-made, spatially imprecise segmentations. This requires a significant mathematical apparatus which is able to cope with multivariate data involving hesitancy, ambiguity and contradiction.
In this talk we analyze, from a historical perspective, the problem of quality evaluation for image segmentation. Specifically, we focus on how to handle the variable interpretation by different humans. This will lead to an analysis of the general quality evaluation problem in the presence of multivariate ground truth, including its semantics, the technical challenges it poses and the relationship with some mathematical disciplines involved in its solution.
C. Lopez-Molina received the Ph.D. degree from the Universidad Publica de Navarra, in 2012, where he is currently an Assistant Professor. His research interests are in low-level feature extraction/treatment for computer vision and automated bioimagery processing. He has developed most of his work around edge and boundary detection, and in soft computing techniques for computer vision.